4 Ways this RSNA Panel Laid Out The Future Of Imaging AI
The paths forward to more rapid and widespread AI adoption in medical imaging.
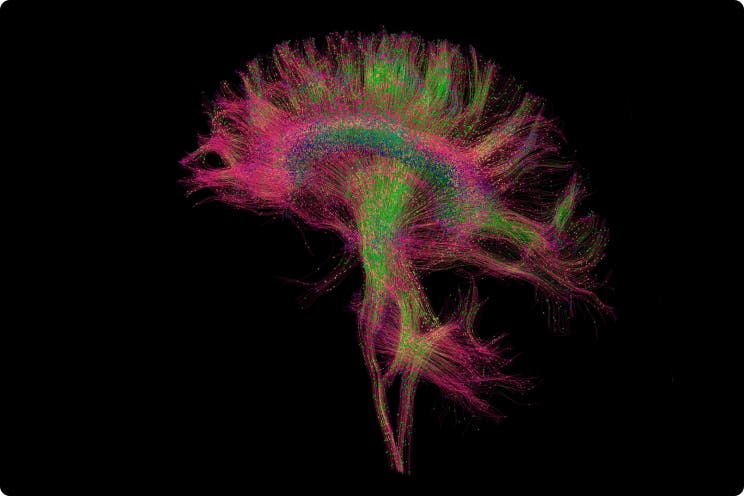
One of my personal highlights of RSNA 2022 was sitting on a panel discussion, co-sponsored by Merative and TeraRecon, that asked the difficult question of whether AI was a friend or foe in medical imaging and healthcare. It was an honor to join an all-star lineup of imaging AI experts – Matt Dewey, chief information officer for Wake Radiology Rex UNC Healthcare; Eliot Siegel, MD, vice chairman of radiology at the University of Maryland; Sinan Batman, chief technology officer for TeraRecon; and Ajay Choudhri, MD, chair of radiology at Capital Health.
One of the main reasons radiologists went digital years ago was the promise and possibility that AI, sometime in the future, could help us make more accurate and timely diagnoses, and in doing so, improve outcomes. But that future is here, and AI adoption is still in its infancy, with the technology far from achieving its full potential. Although there are hundreds of approved algorithms, most practices have deployed few or none in medical imaging, and those that have been deployed remain narrow. AI adoption is slower than expected – but why?
Here are some of the biggest lessons learned – and paths forward to more rapid and widespread AI adoption in medical imaging.
1. If you build it (the platform), they (the algorithms) will come
What are the problems that we, as radiologists, hope to solve with AI? It’s not simply finding lung nodules or pulmonary emboli faster. I believe that a key value of AI is enhancing workflows. Making the data run more smoothly. Creating more user-friendly worklists. Triage and detection algorithms are important, but as stand-alone solutions, they are not making a radiologist’s daily workflow smoother. With increasing image volumes, more complex cases, chronic diseases and a shortage of radiologists to do the work, I believe that creating easier workflows, and the platforms to support them, are necessary.
We’re not going to get anywhere with AI adoption if we’re chasing algorithms for every specific purpose. An AI platform approach flips the script: instead of needing AI expertise to create narrow algorithms from scratch, and asking understaffed and overstretched IT teams to roll out those algorithms at a fast and furious pace, you can rely on AI orchestration to bring the algorithms to you. The platform opens the door to an ecosystem of algorithm possibilities, and the imaging organization’s internal stakeholders can pick and deploy the applications that best address their clinical needs and workflow challenges.
2. Who do you trust on algorithm validation?
How to adjudicate trust in AI algorithms was a major and lively point of debate at our RSNA panel. Reasonable people will disagree – and we did! Some will argue the FDA should have jurisdiction on individually validating algorithms. There are a lot of bad algorithms out there that don’t perform as advertised and the FDA should weed them out. Others will argue that adjudicating vendor trust in algorithms shouldn’t fall to the FDA at all; that in a platform-driven world, algorithms will need to be validated by the platform (in which case, if you trust the platform, you implicitly trust the algorithms it gives you). Or, in the interest of supporting as wide an ecosystem as possible, validation should fall to individual organizations trialing each one, even if it means ‘bad’ algorithms are made available by the platform. IT teams can’t just reflexively trust the FDA, platform, or vendor for this adjudication to be done; they need to perform it themselves. I think we all agreed, however, that platforms and AI orchestrators are a glide path to accelerating AI adoption.
Here's my take: to some extent, I think we’re all overthinking it. Diagnostic reports are signed by the radiologist, not the AI; it’s our name on the interpretation. AI is just a tool, like voice recognition in PACS. We can’t assume these algorithms will be perfect. Integrating AI into our workflows is about improving our workload management. It’s up to us to discern what works and what doesn’t, what’s right and what’s wrong, when to listen to it and, ultimately in each of our practices, how we can use it to improve our lives and outcomes for our patients.
3. AI is neither ‘friend nor foe’
Ultimately, AI is neither a radiologist’s best friend nor worst enemy – it’s simply a technology tool. It can transform workflows for the better for those who know its strengths and it can be a pitfall for those who don’t understand its limitations. At the end of the day, AI is what we make of it.
As radiologists, unfortunately, on average, we only spend about 15% of our time actually doing what we are trained to do: looking at images and making diagnoses. The other 85% is spent on arranging images, looking at reports, parsing worklists to find the next study in the queue and being interrupted by other distractions. Our goal as an industry – for the sake of our patients and for our own mental health – must be to keep increasing that 15%. And I believe AI is one of the most immediate and untapped pathways for getting there.
It’s easy for skeptics to call out when an algorithm errs as an example of why the whole technology must be dismissed or ignored. But why hammer on an algorithm’s miss rate and ignore the human rates of attrition among radiologists? Or our well known 3-5% error rate? Or the 50% burnout rate among radiologists that is rippling to a point where it affects our patients, too. It’s not unimportant if an AI algorithm misses a lung nodule, but that’s a reason to keep refining AI, not to drop it altogether.
4. Even the skeptics agree: AI-infused and cloud-first workflows are here to stay
Whether you’re all-in on cloud-native or are still a cloud skeptic, we all agreed: everything is going to go on cloud. Within the next 10 years, sending data up into the cloud and receiving back outputs from different algorithms is going to be in the DNA of how radiology functions. With the exponential volume of data and complexity of diseases we’re facing, using the cloud as part of our workflows to distribute workloads and valuable AI insights is a proven and compelling way for solving this workflow problem.
That means better and faster imaging. More efficient and productive algorithms. Trends-based predictions made by algorithms studying prior studies. Algorithms that can optimize themselves for their specific institutions and make predictions tailored to those populations. And perhaps most importantly, a transformation from narrow to broad AI, expanding beyond looking at individual nodules to tracing entire family histories and community health profiles to make more informed patient care decisions. Including not just the genetic code, but also the zip code. And the list goes on.
The combination of AI and cloud will help usher a shift from focusing on individual algorithms to the best possible combinations of AI-infused workflows. At the end of the day, it’s not the algorithm, it’s the workflow. So long as AI fits into your workflow, isn’t intrusive and helps drive better results when reading studies, radiologists will accept it as neither friend nor foe, but as another critical tool in their arsenal.
Related Articles
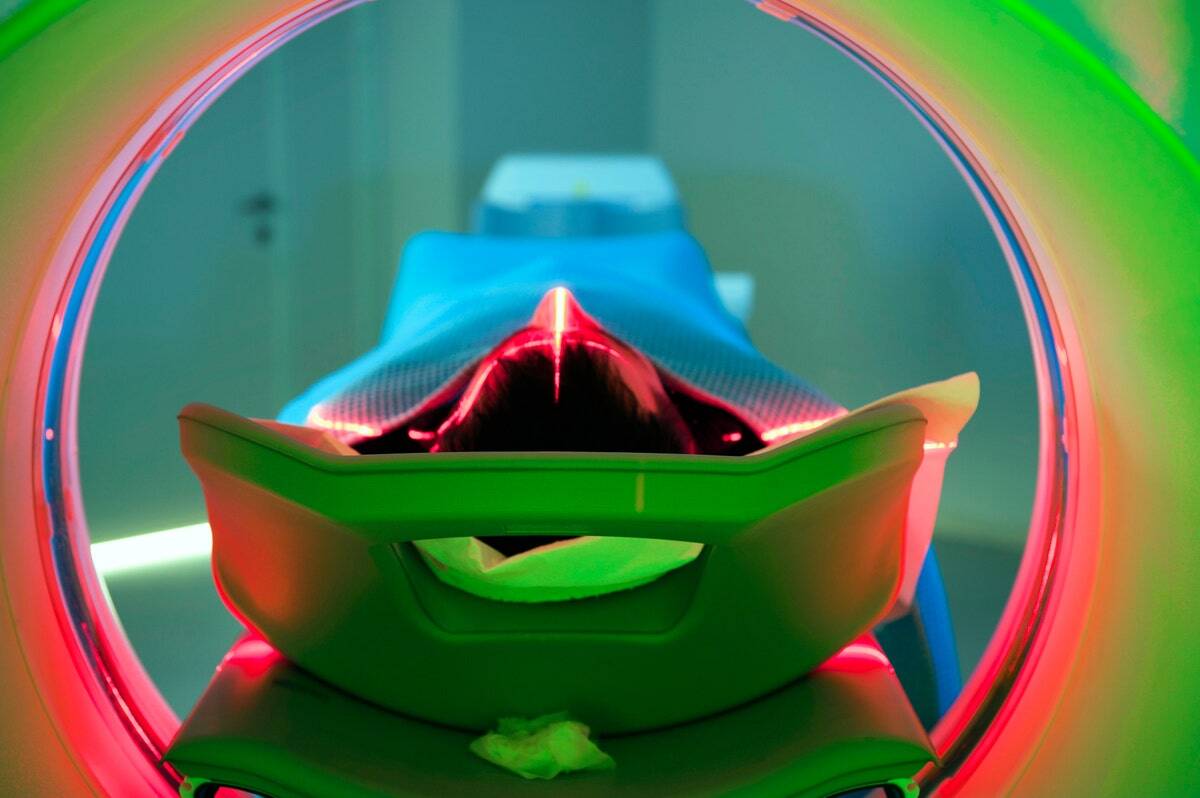
The 4 imaging AI questions we all need to be asking
By David Gruen | 6 min. read
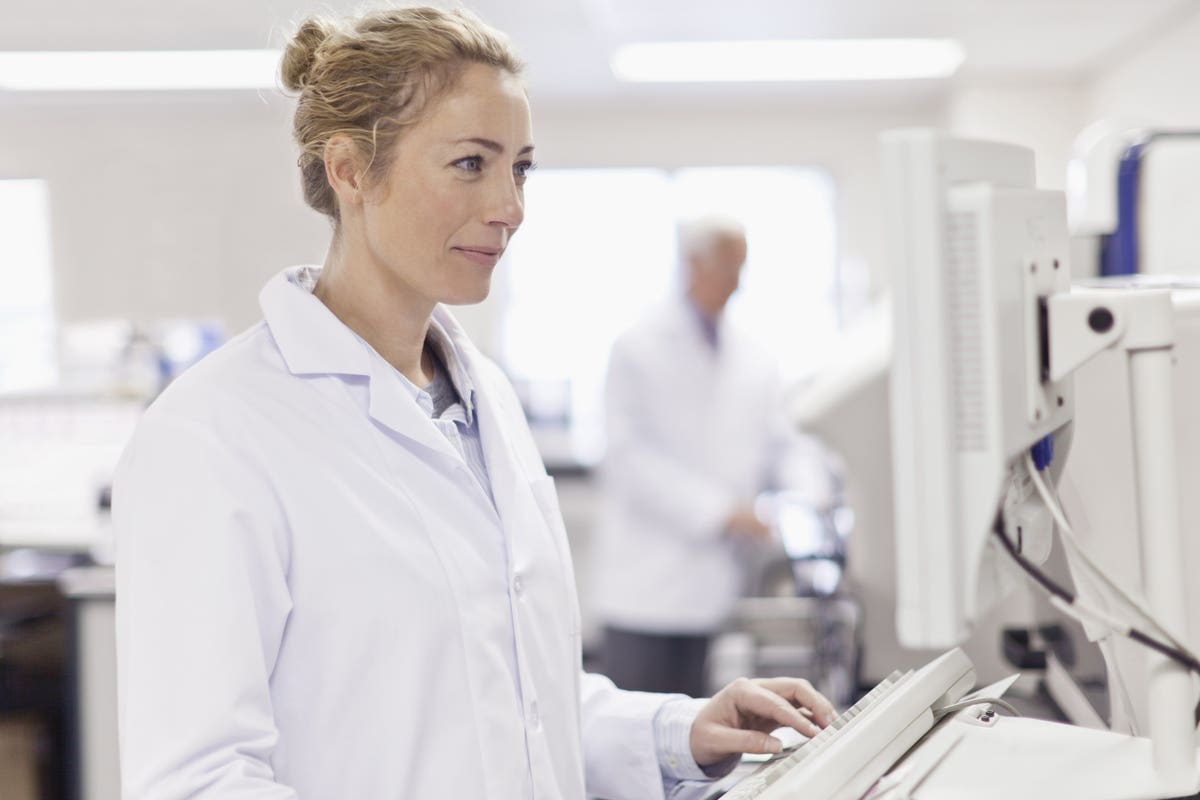
Beyond KLAS rankings: building client relationships at Merative
By David Gruen | 3 min. read
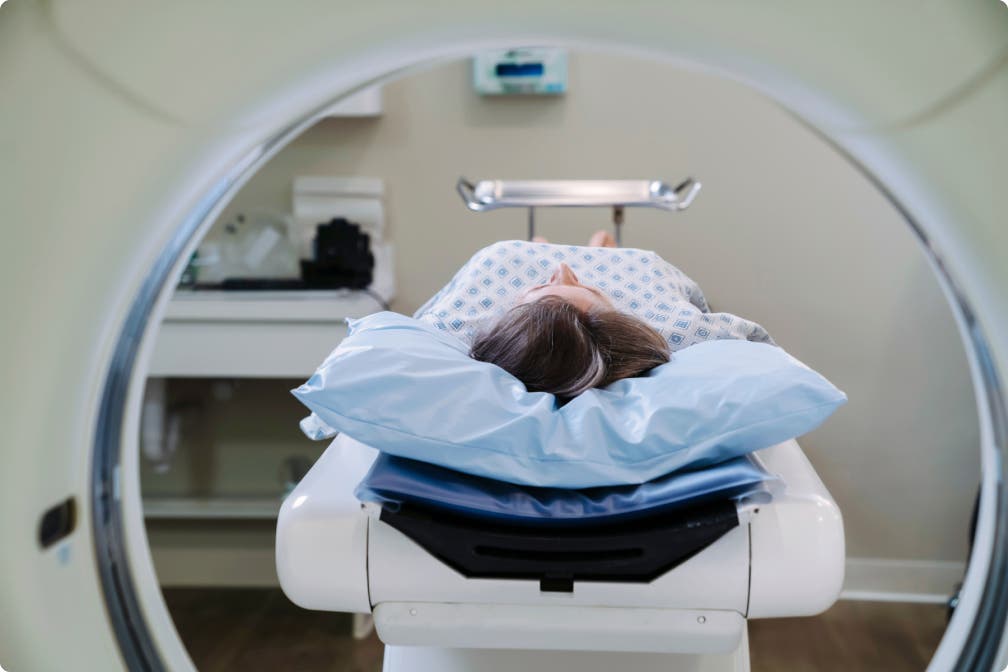
Merge October 2023 newsletter: Keeping patients at the center
October is Breast Cancer Awareness Month, a topic that is important to me personally...
By Julie Pekarek | 4 min. read
Ready for a consultation?
Our team is ready to answer your questions. Let's make smarter health ecosystems, together.